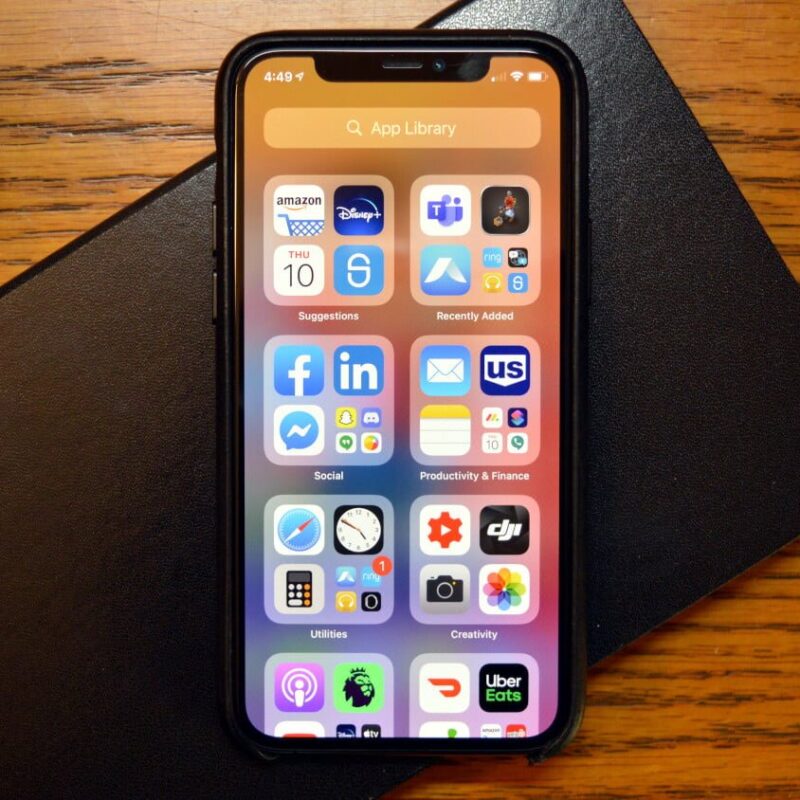
iOS interview questions
Top 35+ iOS Interview Questions and Answers for 2024
This article lists some of the most commonly asked iOS interview questions for freshers and…
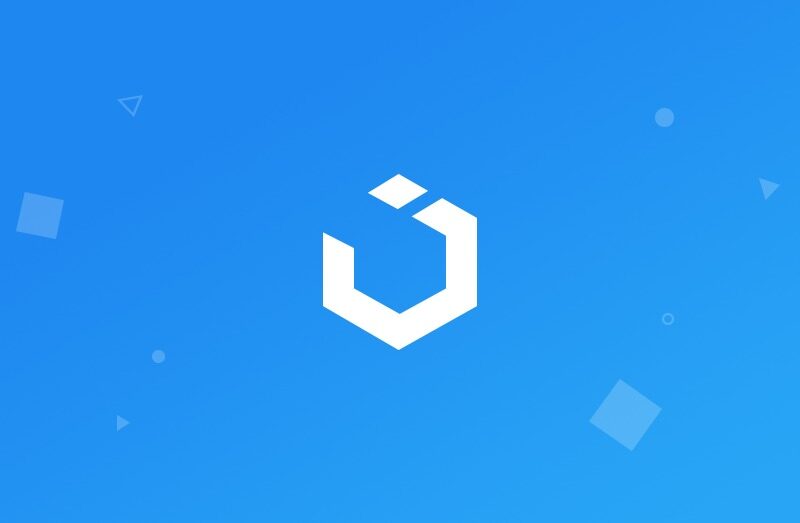
iOS App Design
iOS Interview Questions (UIKit)
UIKit is a framework that was introduced by Apple in 2007 alongside the original iPhone.…
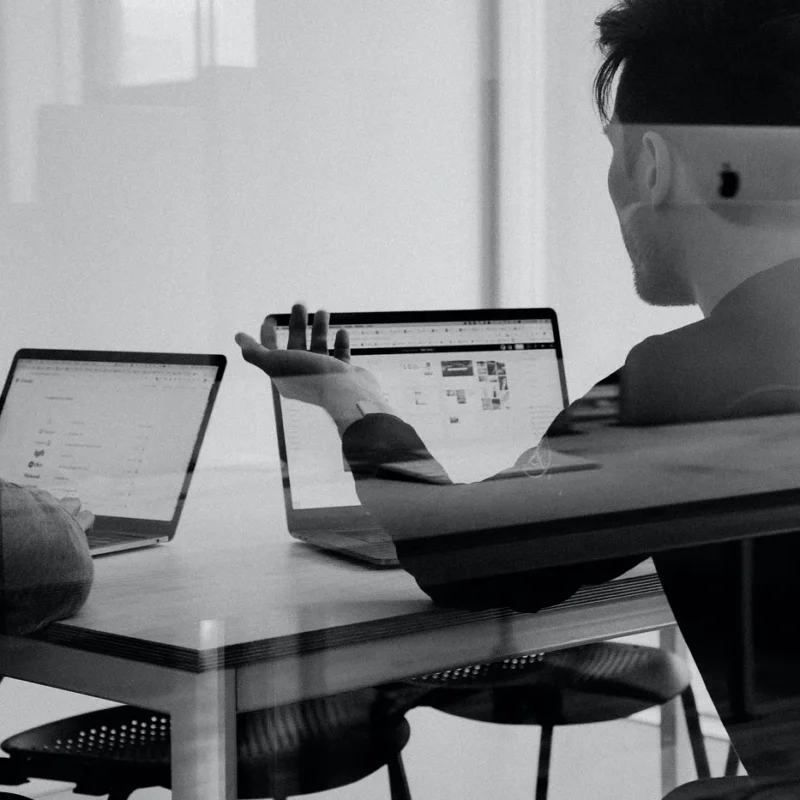
SwiftUI
iOS Interview Questions (SwiftUI)
The existence of SwiftUI has somehow rendered UIKit obsolete, you should get busy learning SwiftUI because it…
Recent Posts
Recent Comments
No comments to show.
https://www.youtube.com/watch?v=W7YKO3t30Rc